Clinical interoperability, or the sharing of information across health systems, has become a buzz word in today’s world. Consider a typical patient who receives health care from their primary care doctor, sees a specialist to whom they may be referred, then makes an emergency room visit to a local hospital. The patient now has three separate medical records in three different systems.
Since patient data is not always shared amongst various systems, one practice or provider may not know of the patient’s existing conditions that are stored in another practice’s system, resulting in the possibility of inappropriate treatment or a missed opportunity for providing holistic care and follow-up. In the age of COVID-19, it is especially important for a provider to know if their patients have had a COVID-19 related diagnosis at another practice and what treatments they may have received. The value of linking clinical data from disparate systems is very high—and to anyone in the healthcare field, instinctively obvious.
To help solve these challenges for our customers, Azara Healthcare has developed its own Master Patient Index (MPI) that matches patients across multiple systems based on patients’ Demographics, Health Plan, and/or HIE data. Our process combines matched patient’s data into one “Consolidated Patient Record” which can be leveraged in DRVS. Effectively, we’re building a comprehensive dataset from all data sources linked through the MPI.
Below is a visualization of an example Consolidated Patient Record. The graph shows the number of patient records (circles) that make up the Consolidated Patient Record and the relationship (lines) between two circles. The table below displays the details of how the two circles are matched, with circle color coordination between the graph and the table.
At a very high level, an MPI follows the following process.
- Data is ingested from multiple sources.
- A list of matching rules is applied against patient records to identify matched patients, forming a Consolidated Patient Record.
- The list of Consolidated Patient Records, with data from multiple data sources, is then sent to DRVS.
The MPI matching process takes place behind the scenes. Below is an exploratory graph which shows what an MPI may be made up of. There are four data sources in this example. Each data source is represented by color shades along the perimeter of the circle. The largest is Data Source #1 (purple), containing a total of 5,240 patients. From each data source, we can see bridges of color flowing into other data sources. The larger the bridge, the more patient matches between the two sources there are. The bridge between Data Source #1 and Data Source #3 (orange) contains 3,284 matched patients out of the total of 5,240 patients. That’s 3,284 matched patients unlocked for our users!
How do we validate and improve our MPI? In addition to internal review of matched patients, we leverage valuable feedback from our customers. There is also an opportunity to utilize machine learning to further improve our matching algorithm. As a result, the more MPIs we create and maintain, the more accurate our MPIs become.
We are excited and proud of the MPI system we have developed at Azara and look forward to continuously improving our matching algorithm to deliver the most accurate MPIs to our customers.
Related Articles
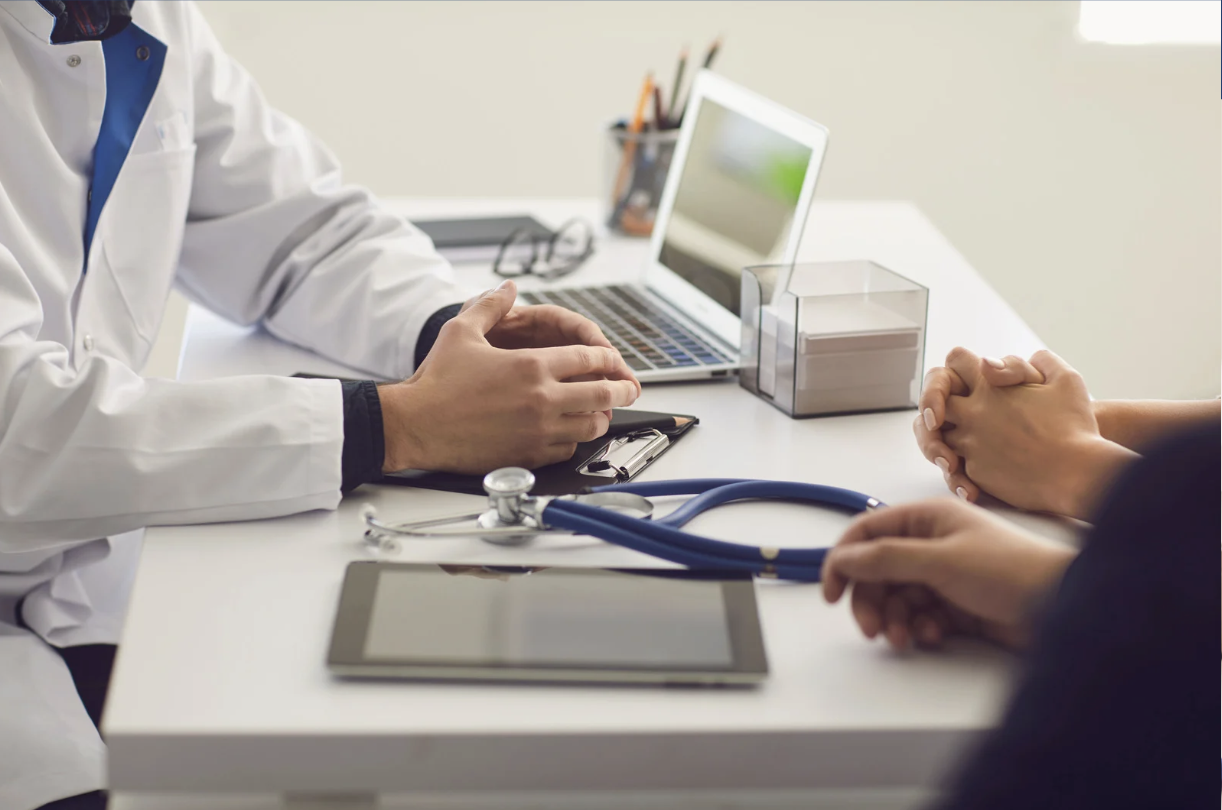
Expanding Care Access Without Increasing Staff: How FQHCs Can Use Data to Work Smarter
Explore Insights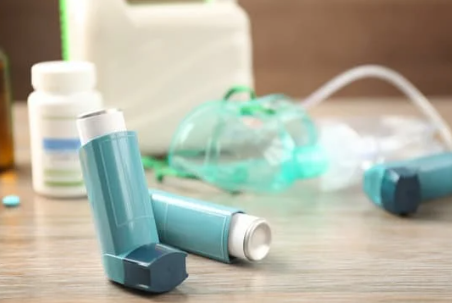
Asthma Awareness Month: Turning Insights into Impact with DRVS
Explore Insights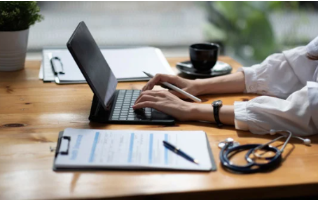